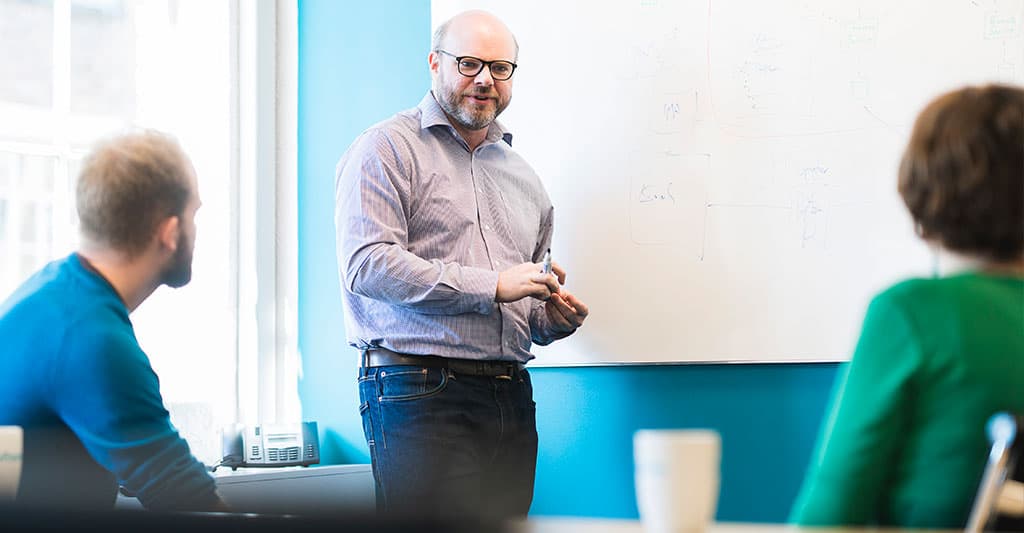
We recently ran a breakfast briefing on artificial intelligence, with Greg Hawkins (former Starling Bank CTO), John Clarke (formerly of Mind Foundry, Nokia, Tesco, and current non-executive director at Ordnance Survey, the DWP and others) and Gareth Edwards (Head of Data Science at Softwire).
During the session, all three gave some really fascinating insights into where AI is today and some of the challenges they’re seeing in organisations they work with. We wanted to share some of these with you, to help accelerate your own use of AI.
1. AI is something you can use today
AI isn’t sci-fi: it’s already transforming the way organisations operate and how they interact with their customers. Banks and other fintech businesses are using AI for biometric authentication, to improve fraud-detection and for risk-analysis, such as deciding whether to approve someone’s credit application. Elsewhere, there are AI-driven security software services that provide increased protection against viruses, malware and inadvertent (or malicious) distribution of sensitive information. We also explored ways in which AI is opening new opportunities in process automation, including the use of Robotic Process Automation to drive automation of legacy systems without the need
for costly interface changes. These are all things you can do today.
2. Similar off-the-shelf vs bespoke trade-offs apply both to AI and conventional software
With AI being a highly specialised field, many of its current applications are being driven by highly specialised vendors. Their products and services are then being adopted by other organisations, such as banks.
The vendor-led approach has been more prominent up to now and can offer some benefits in terms of reduced up-front costs and the ability to leverage a wider pool of data. However, as AI techniques and tools mature, targeted AI applications are becoming practical and affordable, and offer the opportunity to deliver value in a much wider range of situations that are unique to individual businesses.
3. Getting the right data in the right format is still a huge challenge
Many data scientists, hired for their AI expertise to spearhead transformational data-driven projects, typically spend a lot of time chasing around their business to find and collate data. They then spend even more time getting it into the right format to feed into their AI algorithms. Neither is a particularly good use of their time, so it’s essential organisations work towards addressing broader data issues or working with partners who can do the heavy lifting and data-manipulation, to free the skilled data scientists up to apply their expertise where it can be most powerful.
When it comes to incorporating AI into organisations’ ways of working, you may find your biggest hurdle isn’t technology or even data. Instead, it’s the need to change people’s thinking. Individuals have long craved certainty: When you start a traditional tech project, you know (more or less) what the outcomes will be. Equally, when you feed a particular input into a conventional system, you know you’ll get the same output every time. AI and AI projects are different: instead of being about certainty, they’re about probability. Results may not always be what you expect. AI itself may not even provide the answer. Organisations need to embrace this new way of thinking, and not be put off if AI doesn’t end up solving the first problems they try to tackle.
5. Understand what today’s AI can – and can’t – do
So much has been written about AI that it’s sometimes had to separate the facts from the hype: what is today’s AI actually capable of? Gareth Edwards explained the current sweet spot for AI as being tasks that are easy for humans to carry out, but challenging for traditional computer algorithms to tackle. He outlined a project we’re working on at the
moment, using machine learning to extract useful data from till receipts. This work has traditionally been done by a team of people, because conventional computer algorithms haven’t beconventional computer algorithms haven’t been
capable of interpreting the many elements on differently laid-out receipts. Using AI dramatically speeds up the process, reduces errors and means the people can spend more time on higher-value work. The use of AI to screen eye scans for early signs of disease, as trialled by DeepMind and Moorfields Eye Hospital, is another example. Here, AI was able to interpret eye scans as accurately as world-leading doctors. The consequences of this for eyecare patients could be transformative, including quicker diagnoses (because they needn’t wait for scans to be screened by clinicians) and faster access to treatment (because the clinicians can spend more time treating patients).
6. Don’t start by looking for AI projects. Focus on business needs
With any new technology – whether that’s AI, blockchain, the IoT, or something else – it can be tempting to decide you want to ‘do an AI project’, rather than thinking about the value to your organisation. Doing so is akin to putting the cart before the horse. Instead, assess where you need to improve your business. What’s costing you money, exposing you to risk, or affecting your customer experiences? Focus on addressing these issues using whatever means are appropriate, and bring in AI if your challenge lies in the sweet spot we discussed above.