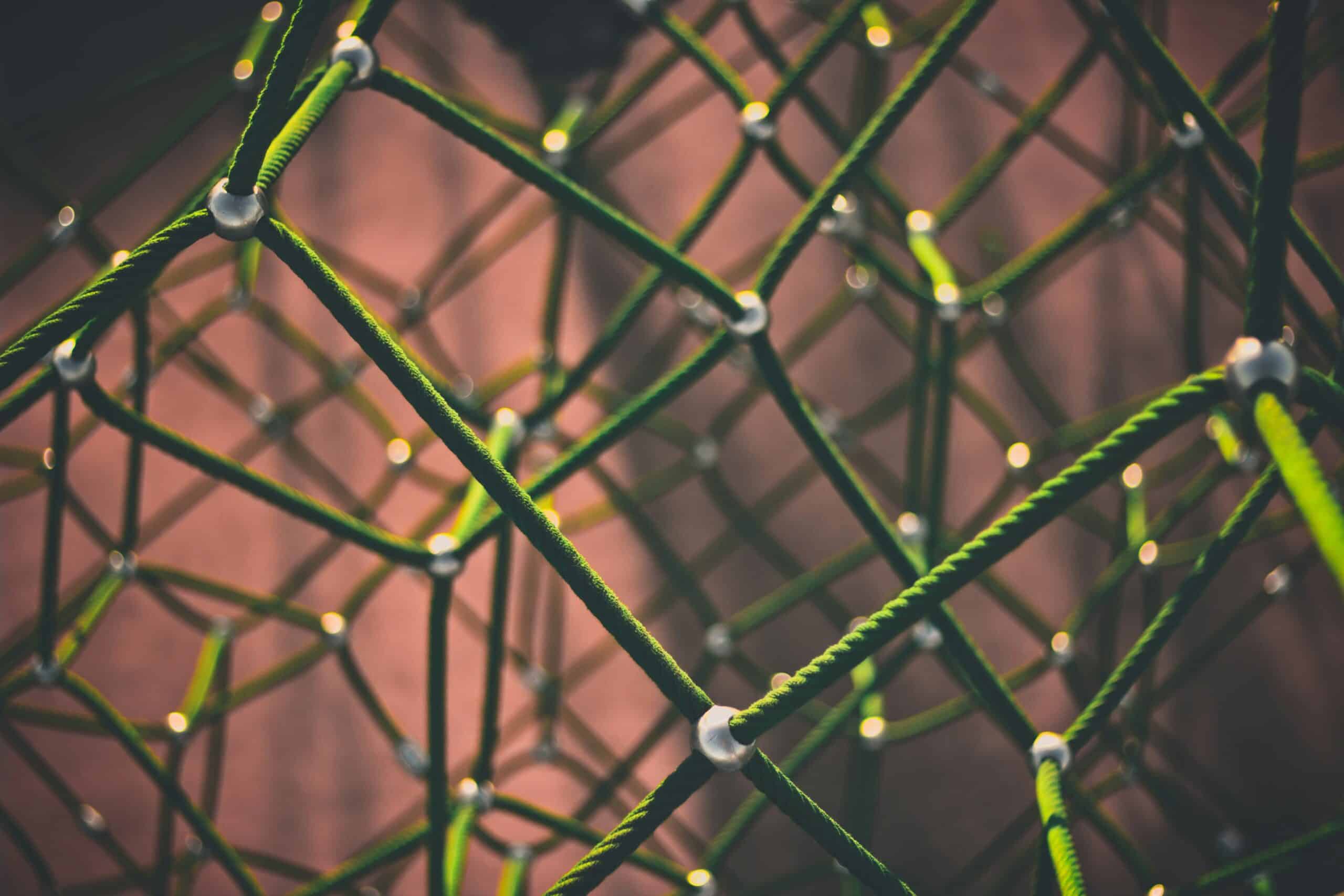
At the start of my PhD, I was told to run to some simple experiments. They were to quantify measurement noise in a variety of conditions, and we didn’t think there would be much to learn. It was only going to take one or two months, which is almost nothing on the usual timescale of academia.
Then one set of measurements yielded something unexpected: a large increase in noise that only appeared under very specific conditions. It would have been easy to dismiss – nanoscale ionic currents are notoriously temperamental – but I measured it again, and I observed it again.
This piqued our curiosity, and thus began two years of work which formed the core of my research, yielding two published papers and multiple offshoot projects. If I hadn’t let the unexpected observation tell me what to look for, that research never would have happened.
Science innovation and business innovation follow the same patterns
The slow pace of scientific discovery is often anathema to business innovation. I was once rejected at the final stage of a job interview because a senior partner thought that academics couldn’t learn to work quickly enough for their firm.
Fortunately, I now work for Softwire, a wonderful digital consultancy who highly value diversity of background. Having been a part of both the scientific and commercial worlds, I can confirm that the process of discovery and innovation is universal and applies to both. Put simply, you start with a hypothesis, then measure an observable which will depend on whether the hypothesis is true or not and see what answer you get.
For instance, your scientific hypothesis might be “this ionic current noise is related to the salt concentration”, and your business hypothesis might be “the customer click-through rate will be higher if we move this button”. In the former case, you measure the noise at various salt concentrations and see how it changes, in the latter you measure the click-through rate for several different button placements and see how it changes. The process is the same, regardless of the setting.
Technical details aside, the universality of these principles is key to how organisations should approach data work. It is a journey of discovery and of research, not of question answering. For instance, Softwire recently did some work for a government department, trying to increase the uptake of a certain service they provide. The assumption was that people didn’t know about this service and that the communication needed to be improved. However, it rapidly became clear that the assumptions were false: people’s first port of call was the government website, but the service itself was too painful and second or third parties refused to engage with it. The more information we gathered, the more it became clear we weren’t asking the right question to begin with.
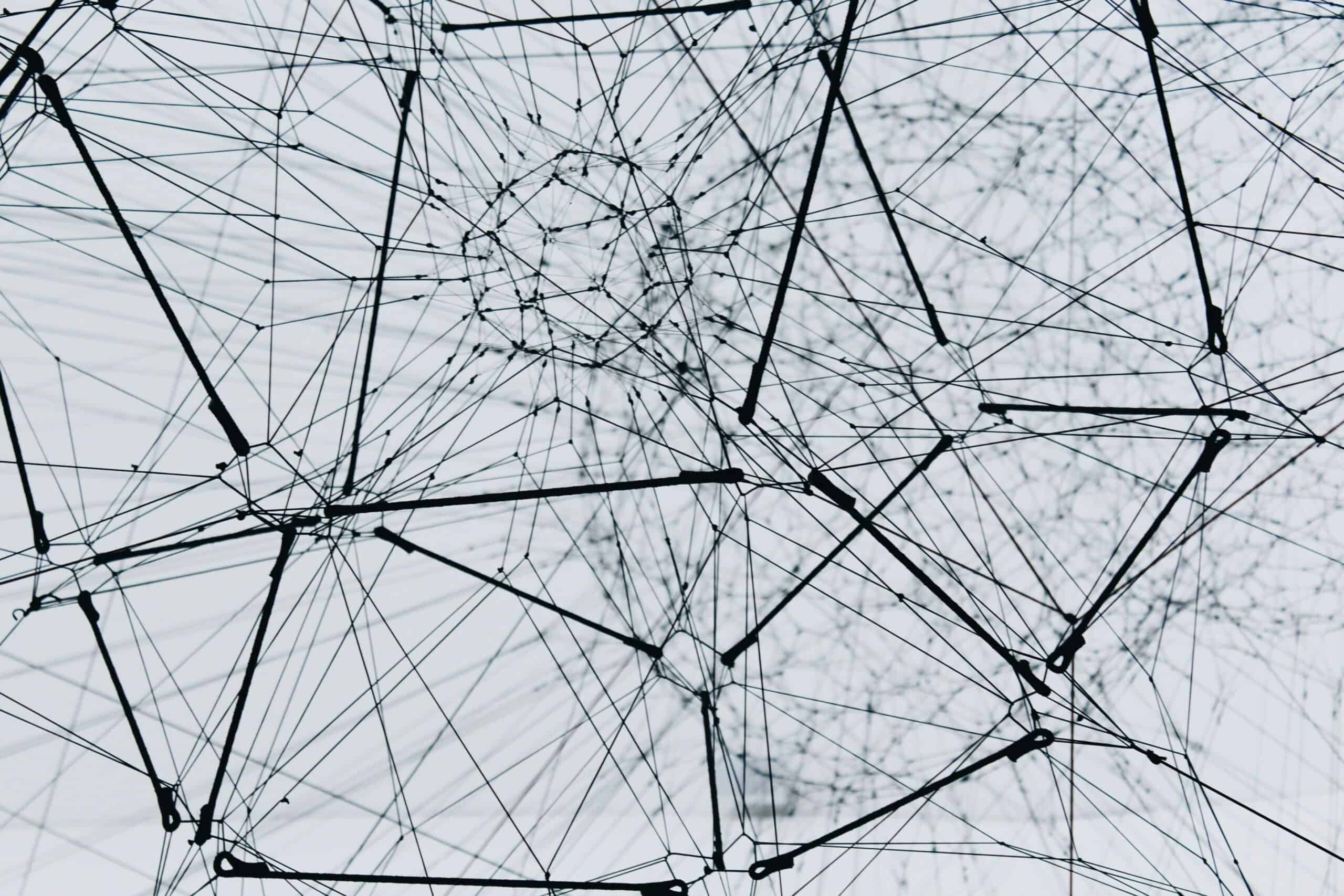
Data is a product of the whole system, not just the bit you’re measuring
When you measure something about a complex system, the results are a consequence of the whole system. A user’s choice to click the button will be related to your UI, without a doubt. However, it may also be impacted by whether the user has their browser on dark mode, or whether they’re hungry, or tired, or what website they visited just before yours. When all you can measure is whether a user clicks a button, all these effects get implicitly averaged out, but they’re still present: uncountable inputs, all boiled down to a single data point.
Sometimes, however, they may not average out. If you don’t explicitly control for an input variable then the output may have dependence on it. You may notice something unexpected in your data. I did.
This is the most valuable type of observation since it raises a question. When your question comes from your own mind it’s a product of all your preconceptions, thoughts, assumptions, and biases. When your data raises a question, it has none of those.
I was working with a client recently who assumed that their users would always want the most up to date documents and information possible. We noticed, however, that most users had two to four old reference sources that they repeatedly used in addition to the new material. We learnt that familiarity was a major component of how the users could find information, so we tailored our search service to account for that.
Openness to the scientific method requires willingness to pull on threads that may or may not yield results. This is all an admittedly roundabout way to describe the process of research, but I want to emphasise the most often neglected part of the process: curiosity.
If data is viewed as an answer factory, then you won’t have time to be curious. Instead, think of data as a window into a living, breathing system – not just a bunch of metrics. Then you’ll be in the best position to spot unexpected and interesting patterns.
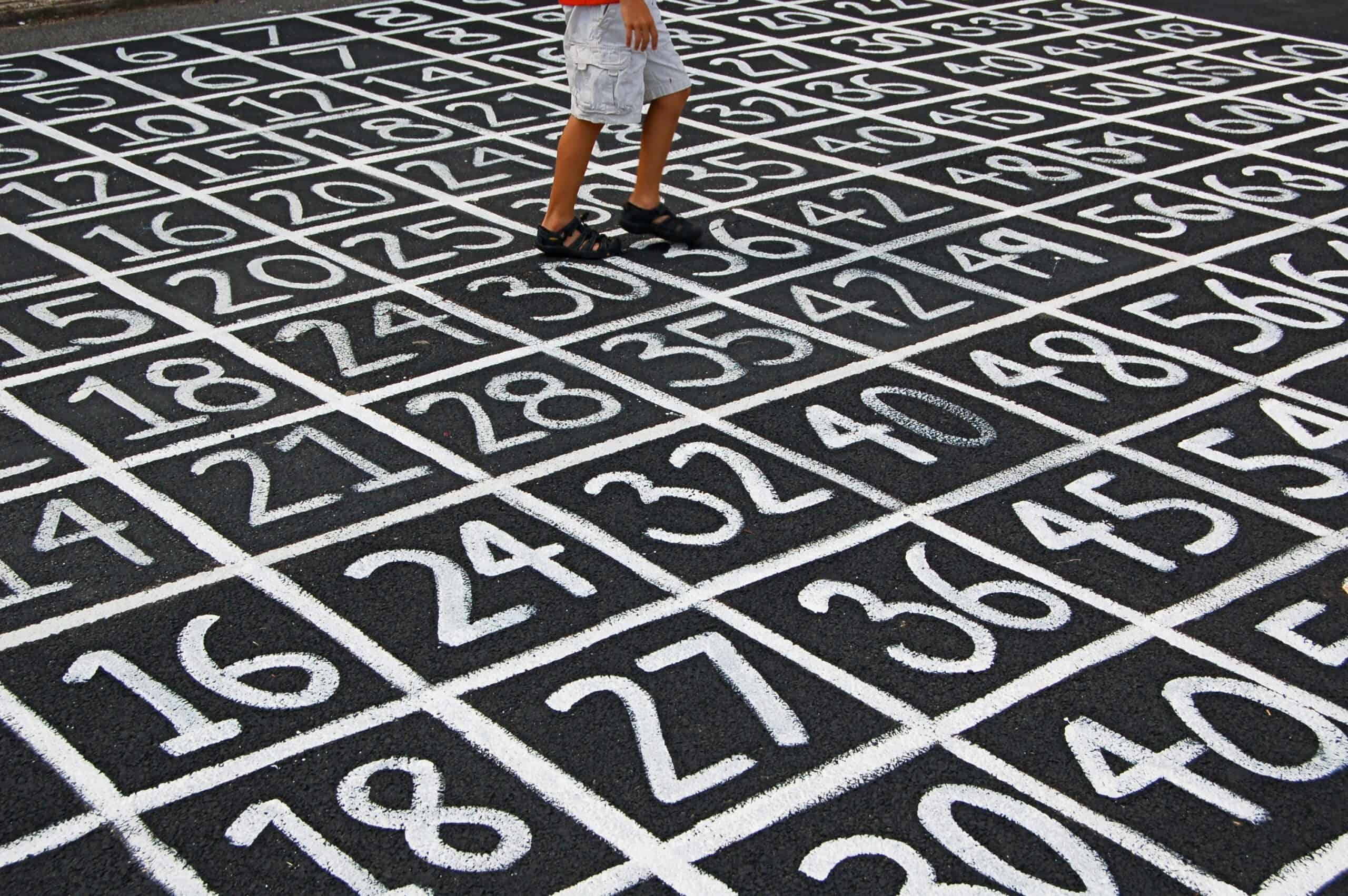
Spurious correlations aren’t scary if you remember to test them
This approach may ring alarm bells – everyone’s heard at some point that correlation doesn’t imply causation. But refusing to let your data pose the questions because of this is throwing the baby out with the bathwater. Fortunately, this problem can be avoided with two simple questions: is the pattern interesting and can you find a root cause?
If a pattern is not interesting, then you need go no further. I don’t mean intellectually interesting, but whether it’s useful to know the answer. If you realise that 70% of the time you put your left sock on first it’s a pattern, but you’re not going to get better at dressing yourself by finding out why.
Being able to find a root cause is the stricter requirement. Using data to provide your questions does not excuse you from forming reasoned hypotheses, so when you spot a pattern, you need to think about why it could happen. Once you’ve done that you can design a more systematic investigation to test that reasoning.
This is not a brand-new process, it’s simply a new step at the start of the process. Your data’s showing you the question, but you’re verifying the answer as thoroughly as you would any other.
Conclusion
Your data is more than just numbers, it’s the shadows on the wall of Plato’s Cave to the system it comes from. It contains fundamental information, however it might not always be obvious exactly where.
That’s scientific discovery: inferring the truth from multiple incomplete pictures, and incrementally expanding your knowledge.
Business data is not different from scientific data in this regard. So be curious. Don’t just ask questions and use data to answer them, ask questions about the data. Find the hidden patterns, bring them into the light, and investigate the things you wouldn’t even think to ask about. You never know what you might learn.